End-to-end behavioral mode clustering for geosynchronous satellites
A new paper from researchers in the Astrodynamics, Space Robotics, and Controls Laboratory (ARCLab) shows that AI can be used to understand satellite behavior from data collected via passive observation. The ARCLab algorithm can identify much smaller-magnitude differences in on-orbit activities, correctly labeling differences in propulsion technology and station-keeping strategy without being exposed previous examples of such behaviors.
Authors: Thomas G. Roberts, Victor Rodriguez-Fernandez, Peng Mun Siew, Haley E. Solera, Richard Linares
Citation: 24th Advanced Maui Optical and Space Surveillance Technologies Conference (September 19-22, 2023)
https://www.researchgate.net/publication/374025335_End-to-End_Behavioral_Mode_Clustering_for_Geosynchronous_Satellites
Abstract
Unsupervised machine learning techniques can be used to describe historical satellite behavior in the geosynchronous orbital regime with higher fidelity than the colloquial terms most often used by satellite operators and the space situational awareness research community. Although satellite behavior is often described with a handful of shorthand operational modes-east-west station-keeping, libration orbits, and retirement drift in the graveyard orbit, for example-more detailed behavioral modes that differentiate station-keeping maneuver frequencies, magnitudes, and propulsion type can be identified via machine learning. This paper contributes to the GEO satellite pattern-of-life characterization literature in a novel way: dissecting historical GEO satellite position time-histories into short segments and sorting them into groups of similar features using time-series k-means clustering. Once sorted, the populations within each cluster can be analyzed further to uncover similarities that may be differentiating observed behavior, including the type of propulsion mechanisms on board and the age of the satellite bus. Unlike previous efforts in behavioral mode clustering, the algorithm presented in this work can be deployed on long-duration satellite position time-histories across many different behavioral modes, with a single learning step from input to output (“end-to-end”). Additionally, there is no requirement to first identify pattern-of-life (PoL) nodes, in which on-orbit behavior drastically shifts from one behavioral mode to another; instead the presented algorithm can identify segments that contain PoL nodes, effectively identifying the nodes themselves as part of the process.
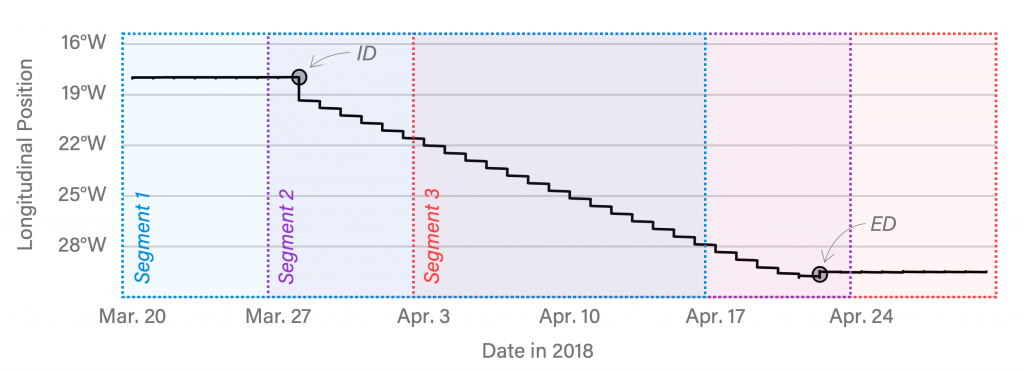
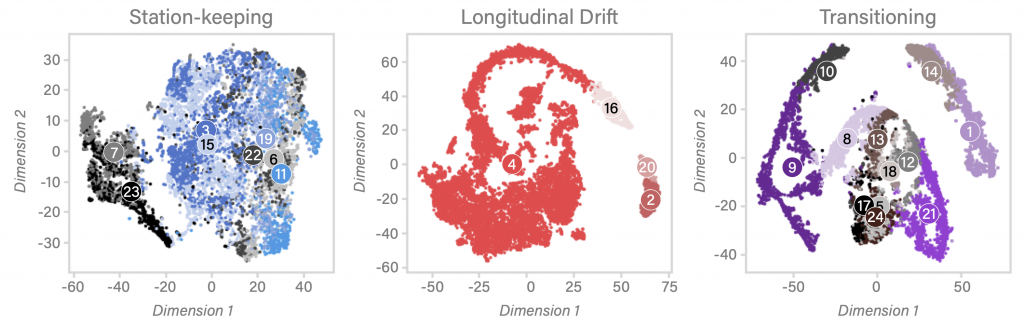